Quick Start¶
In this introduction notebook we provide a simple implementation of a Bayesian Logistic Regression (BLR) model so that users can become familiarized with our library and how to implement their own computational statistics models.
First, we begin by importing pyHaiCS
. Also, we can check the version running...
import pyHaiCS as haics
print(f"Running pyHaiCS v.{haics.__version__}")
Example 1 - Bayesian Logistic Regression for Breast Cancer Classification¶
As a toy example, we implement below a classic BLR classifier for predicting (binary) breast cancer outcomes...
import jax
import jax.numpy as jnp
from sklearn.datasets import load_breast_cancer
from sklearn.model_selection import train_test_split
from sklearn.preprocessing import StandardScaler
# Load the breast cancer dataset
data = load_breast_cancer()
X, y = data.data, data.target
# Split the dataset into training and testing sets
X_train, X_test, y_train, y_test = train_test_split(X, y, test_size=0.2)
# Standardize the data & convert to jax arrays
scaler = StandardScaler()
X_train = jnp.array(scaler.fit_transform(X_train))
X_test = jnp.array(scaler.transform(X_test))
# Add column of ones to the input data (for intercept terms)
X_train = jnp.hstack([X_train, jnp.ones((X_train.shape[0], 1))])
X_test = jnp.hstack([X_test, jnp.ones((X_test.shape[0], 1))])
First, we train a baseline point-estimate logistic regression model...
from sklearn.linear_model import LogisticRegression
from sklearn.metrics import accuracy_score
def baseline_classifier(X_train, y_train, X_test, y_test):
clf = LogisticRegression(random_state = 42)
clf.fit(X_train, y_train)
y_pred = clf.predict(X_test)
accuracy = accuracy_score(y_test, y_pred)
print(f"Accuracy (w/ Scikit-Learn Baseline): {accuracy:.3f}\n")
baseline_classifier(X_train, y_train, X_test, y_test)
Now, in order to implement its Bayesian counterpart, we start by writing the model and the Hamiltonian potential (i.e., the negative log-posterior)...
# Bayesian Logistic Regression model (in JAX)
@jax.jit
def model_fn(x, params):
return jax.nn.sigmoid(jnp.matmul(x, params))
@jax.jit
def prior_fn(params):
return jax.scipy.stats.norm.pdf(params)
@jax.jit
def log_prior_fn(params):
return jnp.sum(jax.scipy.stats.norm.logpdf(params))
@jax.jit
def likelihood_fn(x, y, params):
preds = model_fn(x, params)
return jnp.prod(preds ** y * (1 - preds) ** (1 - y))
@jax.jit
def log_likelihood_fn(x, y, params):
epsilon = 1e-7
preds = model_fn(x, params)
return jnp.sum(y * jnp.log(preds + epsilon) + (1 - y) * jnp.log(1 - preds + epsilon))
@jax.jit
def posterior_fn(x, y, params):
return prior_fn(params) * likelihood_fn(x, y, params)
@jax.jit
def log_posterior_fn(x, y, params):
return log_prior_fn(params) + log_likelihood_fn(x, y, params)
@jax.jit
def neg_posterior_fn(x, y, params):
return -posterior_fn(x, y, params)
# Define a wrapper function to negate the log posterior
@jax.jit
def neg_log_posterior_fn(x, y, params):
return -log_posterior_fn(x, y, params)
Then, we can call the HMC
sampler in pyHaiCS
(and sample from several chains at once) with a very high-level interface...
# Initialize the model parameters (includes intercept term)
key = jax.random.PRNGKey(42)
mean_vector = jnp.zeros(X_train.shape[1])
cov_mat = jnp.eye(X_train.shape[1])
params = jax.random.multivariate_normal(key, mean_vector, cov_mat)
# HMC for posterior sampling
params_samples = haics.samplers.hamiltonian.HMC(params,
potential_args = (X_train, y_train),
n_samples = 1000, burn_in = 200,
step_size = 1e-3, n_steps = 100,
potential = neg_log_posterior_fn,
mass_matrix = jnp.eye(X_train.shape[1]),
integrator = haics.integrators.VerletIntegrator(),
RNG_key = 120)
# Average across chains
params_samples = jnp.mean(params_samples, axis = 0)
# Make predictions using the samples
preds = jax.vmap(lambda params: model_fn(X_test, params))(params_samples)
mean_preds = jnp.mean(preds, axis = 0)
mean_preds = mean_preds > 0.5
# Evaluate the model
accuracy = jnp.mean(mean_preds == y_test)
print(f"Accuracy (w/ HMC Sampling): {accuracy}\n")
Example 2 - Sampling from a Banana-shaped Distribution¶
In this example, we will demonstrate how to sample from a banana-shaped distribution using pyHaiCS
. This distribution is often used as a benchmark for MCMC algorithms and is defined as follows...
Given data \(\lbrace y_k\rbrace_{k=1}^K\), we sample from the banana-shaped posterior distribution of the parameter \(\symbf{\theta} = (\theta_1, \theta_2)\) for which the likelihood and prior distributions are respectively given as:
The sample data are generated with \(\theta_1+\theta_2^2=1, \sigma_y=2, \sigma_{\theta}=1\). Then, the potential is given by:
The resulting samples were produced for 10 independent chains, each with 5000 burn-in iterations, 5000 samples, \(L=14\) integration steps, a step-size of \(\varepsilon=1/9\), and a momentum noise of \(\phi=0.5\).
First, let's generate some synthetic data based on the description. We set \(\theta_1+\theta_2^2=1\), \(\sigma_y=2\), and \(\sigma_{\theta}=1\). We will generate \(K=100\) data points (provided in the benchmarks), and then estimate the parameter posteriors using HMC or any other sampler.
filePath = os.path.join(os.path.dirname(__file__),
f"../pyHaiCS/benchmarks/BNN/Banana_100.txt")
y = pd.read_table(filePath, header = None, sep = '\\s+').values.reshape(-1)
# Initialize the model parameters
key = jax.random.PRNGKey(42)
key_HMC, key_GHMC = jax.random.split(key, 2)
mean_vector = jnp.zeros(2)
cov_mat = jnp.eye(2)
params = jax.random.multivariate_normal(key_HMC, mean_vector, cov_mat)
sigma_y, sigma_params = 2, 1
# HMC Sampling
params_samples_HMC = haics.samplers.hamiltonian.HMC(params,
potential_args = (y, sigma_y, sigma_params),
n_samples = 5000, burn_in = 5000,
step_size = 1/9, n_steps = 14,
potential = potential_fn,
mass_matrix = jnp.eye(2),
integrator = haics.integrators.VerletIntegrator(),
RNG_key = 120, n_chains = 10)
@jax.jit
def potential_fn(y, sigma_y, sigma_params, params):
return 1/(2 * sigma_y ** 2) * jnp.sum((y - params[0] - params[1]**2) ** 2) \
+ 1/(2 * sigma_params ** 2) * (params[0] ** 2 + params[1] ** 2)
The results for the first three chains, using HMC & GHMC, are shown below:
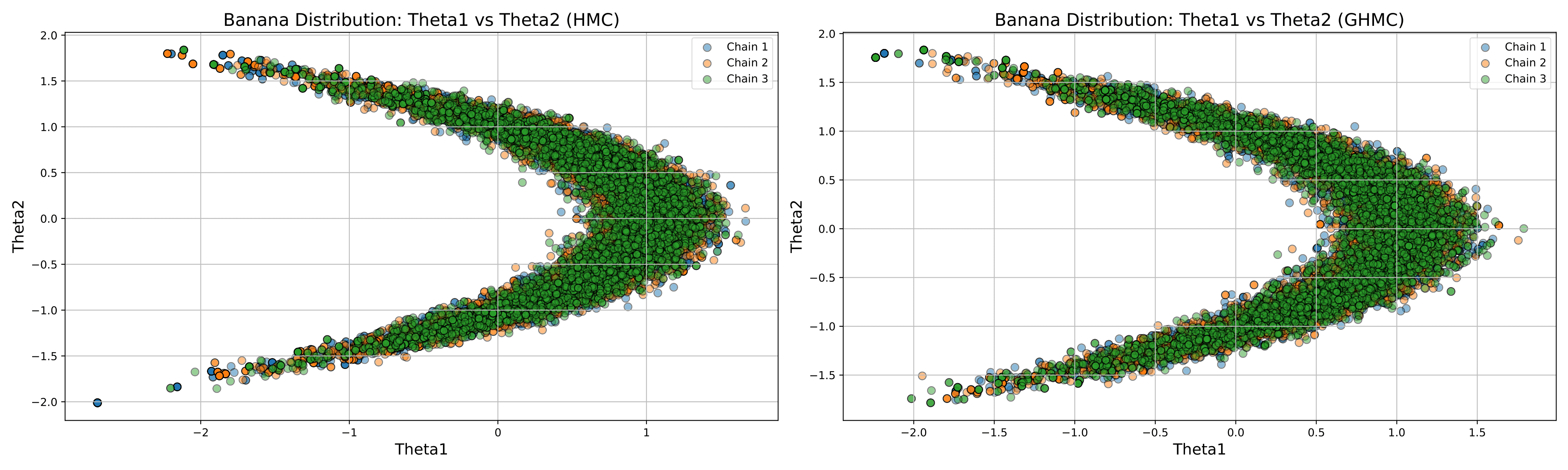